EP 31: A Decade of AI Revolution
Exploring the Evolution of AI from 2012 to 2023
Posted on November 10, 2023 by Fusion Connect
Join us on this captivating episode of Tech UNMUTED as we take a deep dive into the remarkable journey of AI innovation over the past 10 years. From the inception of AlexNet in 2012 to the latest developments in 2023, we uncover the pivotal moments that have shaped the AI landscape. Santi and George dissect the intricate milestones, revealing how AI has evolved to understand, create, and transform industries. Don't miss this insightful exploration of the past, present, and future of artificial intelligence.
Watch & Listen
Tech UNMUTED is on YouTube
Catch up with new episodes or hear from our archive. Explore and subscribe!
Transcript for this Episode:
INTRODUCTION VOICEOVER: This is Tech UNMUTED. The podcast of modern collaboration – where we tell the stories of how collaboration tools enable businesses to be more efficient and connected. With your hosts, George Schoenstein and Santi Cuellar. Welcome to Tech UNMUTED.
GEORGE: Welcome to the latest episode of Tech UNMUTED. Today, we're going to take a look at the history of AI really, last 10 years of AI. Santi found a great article, so I'm going to pop it up here on the screen. This was written by Thomas Dorfer, who's a data and applied scientist at Microsoft. He starts to break down really what's happened since 2012, how we've gotten to where we are today with AI. Santi and I will go back and forth a little bit, but we'll break down some of the elements that are in here. Pop it up. Make it a little bit bigger on the screen. Santi, you want to jump in on this?
SANTI: Oh, yes, man, I love this graph. [chuckles] You know what I love about this graph is that it forces me to have to go back and think about the evolution of things, right? A lot of people actually are probably looking at this graph right now and going, "I never heard of these things." This is a great opportunity. I'm going to do the best I can. Now, listen, fair warning. I am not an AI engineer, but man, I know enough to be dangerous, and so I'm going to try and be as dangerous as I can today.
At the same time, I want to kind of keep it in layman's terms because we want to be able to make this easy to digest. Listen, it's interesting that he starts his article. Well, I will, he specifically said last 10 years, but it's interesting to me that he started with AlexNet. Before I even get into AlexNet, I thought about this. I said, "Unless we explain first what a neural network is, then AlexNet won't make sense." Let me just take a minute here. The term "neural network," neural almost sounds like neurology, right? [chuckles]
GEORGE: Yes.
SANTI: The term "neural network," the best way to describe it is imagine a computer system that is able to literally learn like a human brain. In other words, it's able to recognize patterns and things like images and texts but very similar to how the human brain does it. Hence, artificial intelligence, right?
GEORGE: Yes.
SANTI: Okay, so now that we understand that with that baseline, in 2012, there was a breakthrough that is known as AlexNet. By the way, it was written by a guy named Alex. [laughs] He wasn't very creative. Alex wrote it, so AlexNet it is. What it did is AlexNet, in layman's terms, basically, made computers better at not just recognizing but understanding an image.
George, the last podcast, Sam Husbands, he joined us, and I was with him trying to look at DALL-E and Midjourney. At the beginning of the podcast, he wanted to ask about how computers actually-- do they actually understand and comprehend what's going on? My response to him was, "Yes, they do." They can absolutely look at an image. Not only do they recognize, but they understand what it is. That is a breakthrough that happened in 2012 with AlexNet. That's what that is. That's a great place to start.
GEORGE: It was the training, right? It was the repetitive nature of, "Is this what we think it is? Is this a picture of a dog? Is this a picture of a dog? Is this a picture of a dog?" until it starts to say, "It is." I saw somebody speak a couple of days ago. The analogy they used in those instances was like an art critic. It says, "No, it's not a dog. No, it's not a dog. Maybe it's a dog. It's getting closer to a dog. Okay, it's a dog," right?
SANTI: Right, right, right, right. I love that analogy. Everything on this chart, honestly, it's just about improving the learning process when you really get down to it, right? Every milestone, as I look across this thing, I'm like, "Yes, it's just getting better and better and deeper." Yes, you're absolutely right. That was the breakthrough. This is where the term "computer vision" comes in where the computer is actually looking like literally looking at an image, analyzing and understanding.
Speaking of computer vision, in 2013, that's where computer vision switched to a neural network. AlexNet was that breakthrough. They went then from computer vision to a whole lot more complexity in the learning process. Basically, computers really started to, again, see and understand. That's the keyword because there's a comprehension, but they started to do it more and more like humans.
Again, it's about mimicking the human brain. What's the best way to mimic a human brain? Well, you take a computer and you have it analyzed and process data from real brains. That's how neural networks start to grow and that's how computer vision starts to switch to that neural network. In this graph, Thomas has an acronym that I haven't heard in a long time, VAE. VAE is critical because, again, in the previous podcast when we were looking at DALL-E and Midjourney, Sam asked a really good question.
He's like, "Is it really learning like the artist's approach to art?" The answer is yes. This is what it does. VAE stands for-- it's variation, so variational autoencoder. I didn't write these things, right? [chuckles] Somebody else came up with these names. Basically, what we're saying is it is a creative system. It's part of the creativity of AI where it's able to learn and generate a new version of what's before it or what data it has received.
It is creating new versions, not a replica. That's the key here. The key here is, this is why when we have AI generate an image, it's unique. In this case, what we're saying is, "Hey, take this specific type of art and generate the following." It's able to understand exactly what you mean by type of art, whatever it is that you chose, and it will use that to create something new, a complete different variation. That happened in 2013. Again, another breakthrough with images. That was a big thing.
GEORGE: Again, a lot of this in this timeline right up until really a year ago.
SANTI: Yes.
GEORGE: These are things that if you weren't directly in the AI space--
SANTI: Yes, you weren't exposed to it.
GEORGE: You didn't know any of this was even going on. You heard about AI. You heard about mechanical automation of things and robotic automation, those kind of things, but there was a lot of work that took place that got us to where we are today.
SANTI: Yes, by the way, everybody knows Face ID on your phone.
GEORGE: Yes.
SANTI: What do you think that's using? It's computer vision. This is why it makes you do all these things with your face and your different angles and it's computer vision. It is understanding. When I put on a pair of headsets or when I take off the headsets, the computer knows that it's me. [chuckles] It doesn't matter because we've been using it, but we didn't really know and understand it until-- you're right, until recently where, now, it's become mainstream, right?
Listen, here in 12/2014, he mentioned something called GAN, G-A-N. This is another pivotal moment. By the way, GAN stands for generative. Now, we're talking about generating stuff, so generative adversarial networks. The bottom line is this was a new approach in 2014 that basically allowed a computer to literally generate new content, unique content, unique images.
It did this from existing data, so that was the key, right? It would take existing data and it would generate something new. This GAN network or this approach, this is what became the framework for what today we call "generative AI." You'll hear that term further down the timeline, the word "generative," in a little bit, but this is it. This is that pivotal moment where that started to happen.
Now, we get into 2015. 2015 is all about memory. Now, we got the AIs to look at things differently and to start generating stuff, but we still had to deal with the memory piece of it. Again, we're talking about artificial intelligence. You need memory. There's a bunch of terms on here. There's something called "residual neural networks." Now, we're still talking about neural networks, but residual, it was just basically a smarter way of doing true deep training or deep learning, right?
It was a way for the AI to get better at going deeper. Basically, what they did is they broke things up into more layers. That's what they did. They broke into smaller layers, more layers, but it allowed the AI to learn quicker. That started to speed things up. Residual neural networks made the learning models go deeper and faster. That started to happen in 2015. Now, there's another acronym that also has the letters RNN, but it's not residual. It's recurrent.
Recurrent neural networks was all about memory. It was about getting the computer to be better at remembering things for more extended period of time. This is very similar. I know it sounds real scary, but this is it. Very similar to human long-term and short-term memory. It's exactly the same. In fact, that's what the acronym LSTM stands for, long short-term memory. Yes, artificial intelligence now has short-term and long-term memory.
All this helped the learning process, right? By the way, as I'm speaking to these things, it's overwhelming for me because I play these scenarios in my head and I get really excited about it. These are not things that one thing was not replacing the other. These are building blocks, right? Most of these elements that we're going to cover today are still used. It's just that they just build upon each other, right?
GEORGE: That speed of that build, there's a curve associated with this that got really steep on the upward end over the last-
SANTI: -few years.
GEORGE: -really 12 months, maybe a little bit more than that, right?
SANTI: Actually, George, in the last 12 months, what happened, it became mainstream. We're going to get to the real pivotal moment in a minute here. That's the one that I really get excited about because it completely shook the AI industry. 2015 was all about that memory, improving that memory. Now, it was interesting that Thomas added AlphaGo because I haven't heard about AlphaGo in so long. [chuckles]
In 2016, basically, there was like an algorithm that they took this algorithm and they combined it with some basic machine learning, pass-fail-type thing. They use a technique called, and it's something I haven't heard in a while, a tree search, okay? They combine these three things so that an artificial intelligent can play the game Go. It's a board game. I've never played Go. I don't even know how to play Go.
Basically, AlphaGo became an AI model that was really good, exceptionally good at playing board games. Obviously, they learned a lot of things from this. They took elements from this for large language AI models later on. It was all about getting AI to become exceptionally good at just playing complex board games and winning every single time. Anyway, so that happened in 2016.
George, 2017, this is it. This is the year, and this is why I get excited. 2017, in my opinion, was the tipping point for all things AI, 2017. In 2017, we now have what's called the transformer architect or architecture, right? It's a new approach to architecting AI models and bringing everything together. It was more efficient. It was really a better training model. When I think of transformers, I think about cars that turn into robots.
GEORGE: We're that thing on the electric pole.
SANTI: Oh, the thing on the electric pole, that explodes every 10 years. It just blows up into green sparks and makes a loud boom. No, it was really about architecting the approach to AI so that it required less time to learn, but transformers is what became prevalent for training, ready for this, large language models. Of course, all this vast data that led to where we are today, 2017, was that tipping point with transformers, with that new approach to the architecture.
Guess what? The word "transformer" starts with the letter T. Guess what else has T in it? GPT. A lot of people don't realize what GPT stands for, right? They hear ChatGPT because that's mainstream now. ChatGPT, yes, but what does GPT stand for? By the way, it's also in a word called BERT. BERT also has a T in it. [laughs] That came in 2018. GPT stands for generative pre-trained transformer. Bingo. Let's take all this data now. The last, I don't know, however long the internet has existed, plus every book ever written that was digitized.
Let's just take all this vast data and shove it into this new transformer architecture and see what we get. Voila. [laughs] Generative pre-trained transformer or GPT. It was that time, right? 2017 was that pivotal switch to transformer architecture and then grab everything else that we talked about before about being able to generate stuff using this architecture and you have yourself a model called GPT, which can generate content on the fly. Then here comes Google with BERT just like Sesame Street Bert, right?
GEORGE: Yes.
SANTI: BERT stands for bidirectional encoded or encoder representations of transformers. I don't know. I don't come up with this stuff, man. I'm just trying to tell you what they all mean. Basically, GPT and BERT became the two key players for advancing what we today call "generative AI," right? They have different functions, right? BERT, it's interesting because BERT, the B stands for bidirectional, the AI model is actually able to process text from either left to right or right to left.
That's the bidirectional piece. It's able to look at it in both ways, which I found interesting. Also, BERT is really-- the use case is mostly for Google Docs to do that smart composer inside of Gmail, stuff like voice assistant, all that stuff in the Google suite that's powered by BERT. Listen, OpenAI, keyword "open." GPT, one, it's not bidirectional. It reads text in one direction only, right? Left to right.
It became the go-to for building applications, for generating code, for writing articles, for writing podcasts. This is when we speak about Microsoft Copilot, for example, it is leveraging GPT. That's where it's going to. That's what GPT stands for. That happened in 2018. Also, in 2018, we have this concept. This is, again, mind-blowing to me. We have this concept known as graph neural networks or just graph.
In fact, you've probably heard of Microsoft Graph. What happens in 2018 is computers start to process this data in a way that resembles how things are connected, hence the term "graph." It's almost like if you create a graph of something. Think of pixels in an image or words in a sentence, how things are connected. AI starts to look at things from that perspective. In fact, when we speak about Microsoft Graph, what are we saying? Well, AI looks at it this way.
Text messages, emails, SharePoint repositories, OneDrives, all these things, and starts to connect them together into a visual graph. This is why when you ask Copilot to generate something based on your company's data, it's able to do that because it's able to step back and look at your entire corporate data in a graph and connect things together. Very much like the human brain does.
GEORGE: More quickly, right?
SANTI: Yes.
GEORGE: Not forgetting, we often, "Where's that PowerPoint?" Maybe named it this. How do I find it, right?
SANTI: Yes.
GEORGE: Much more quickly and in a much more accurate way. Not necessarily in 2018, right?
SANTI: Yes.
GEORGE: It advanced substantially since then.
SANTI: Oh sure.
GEORGE: We're absolutely where we're at today.
SANTI: Yes, for sure. 2019 and 2020, between those two years roughly, this concept of what's called self and supervised learning also came about. It's really about computers again learning more and more and more like humans do and processing like humans do. We're now mimicking. Now, we're mimicking the natural way that humans learn but using a computer. That's what it is. Because how do we learn? Well, there's stuff that we do on our own and there's stuff that we're taught.
That self and supervised learning approach, basically, the AI is mimicking even further how we as humans learn. Guess what? It's doing it much, much faster. I read an article recently, which I found interesting. Not recently, it's been a while, but I started to really get into music and how AI is impacting the music industry. Apparently, the self and supervised learning approach to deep computer learning really became very prevalent and had promising results, believe it or not, in audio signal processing.
Apparently, it's a big deal in the music world or the audio world in general. I found that interesting. I can see that because think of a sound engineer or a producer where there's things that maybe they figured on their own, but they didn't learn everything to that job on their own. There was somebody who has 20 years of experience who has a lot of nuances that you can't learn from a textbook came and coached you on something like, "Whoa, that is awesome." Now, he's transferred that knowledge to you. That's what this is doing, right?
GEORGE: Somebody seen yesterday. They released the last Beatles song. It was a song that John Lennon had recorded. I think it was 1977 on a cassette player. The quality of the song wasn't good and they had tried for years to piece it together. Now, with the advent of AI, they were able to piece it together.
SANTI: I didn't hear about this.
GEORGE: Yes, I saw it late yesterday.
SANTI: Nice.
GEORGE: They were able to piece it all together into a song without noise. Got a lot of background noise on the cassette tape. They've integrated all the original Beatles into it and have been able to process it and release it as a single.
SANTI: Really?
GEORGE: Something that you could have had engineers do maybe, but not at the level that it's been done at, but they weren't able to do it or chose not to do it because they couldn't get to the right quality level. Now, with AI, they've gotten it to the point that it's actually a solid put-together song and releasable.
SANTI: That is fascinating. See? Yes, I'm going to look that article up. Plus, we're Beatles fans. Who isn't a Beatles fan? That's good to know. I'm going to look it up. Now, we're coming to the end, right? Here we are. We're at 2021 heading into the homestretch of 2023. Again, it's interesting that Thomas has AlphaFold. AlphaFold is not something that you and I would ever run into. As frontline information workers, this is not something we would use.
In the medical field, it became a big deal. Basically, without getting into too much detail, AlphaFold was an AI system that was able to predict, ready for this, the 3D structure of a protein based on data like what type of amino acids and all sorts of elements and chemical data that would get fed into the AI. The AI would then produce a three-dimensional picture of a protein.
A lot of times, nowadays, when you see some type of a representation of cells or proteins or viruses or DNA, these images are now generated three-dimensional accurately through AI because of stuff like this. It's big in the medical and biological research realm for sure. That happened in 2021. Here it comes. Now, this is the stuff we all love. In 2021, GitHub Copilot was launched.
GitHub is a developer's platform for Microsoft and developers start using Copilot in 2021 to write their code. [chuckles] It turned the entire programming world on its head because, now, you could be the best developer. Great. With Copilot, you became even better. I read an article recently that they interviewed a bunch of users within GitHub and there was no negative comment whatsoever on Copilot.
They absolutely loved it. They found themselves to be more productive. A lot of these developers are independent contractors, so they were able to finish jobs quicker and move on to new jobs and making more money. See, that's what's nice about this. It's like you were thinking, "Oh, well, Copilot's going to replace developers." No, they just became better at what they do and they did it faster and were able to do more. I found that very, very interesting.
GEORGE: We've talked about that a lot, right? There's two layers. One I'll bring up that we haven't really talked about before, but people were like, "Is AI going to take my job?" The answer to that is the person using AI might take your job, right? AI itself is probably not going to take your job.
SANTI: It's a good point.
GEORGE: The same holds true for corporations. There is a window of opportunity now where companies who take advantage of AI take advantage of Copilot, take advantage of some of the other tools in particular in the Microsoft platform will be more successful than those who don't. In fact, at the margins on that, there's likely a binary outcome. There will be organizations who, by strategic choice, don't use it or lack of capability or lack of funding to implement it will not use AI, or will not leverage it in a significant way. They are going to be the losers, right? Somebody else will gain market share. They'll become more efficient. They'll become more productive.
SANTI: If you want to compete, you got to go embrace AI. That's the bottom line. I keep saying that. By the way, the GitHub Copilot, that's where it started, but it is the same Copilot we speak about. This is the Microsoft Copilot. In other words, now, we know that Copilot is in Bing. They rolled it out to Windows 11. As of a couple of days ago, November 1st, they went ahead and rolled it out to the enterprise customers in their Microsoft 365 suite.
It's going to roll out in the Microsoft phone system in the first quarter. It's just going to keep going. There is no stopping this train. Copilot is going to be how we do work moving forward. Yes, we have to embrace it. We have to learn it. Of course, that same time period of 2021 across 2023, DALL-E came out. This is, again, OpenAI. Same people who own GPT. They have DALL-E.
It generates images based on text descriptions, right? This is what we did in the previous podcast where we pinned DALL-E up against up against Midjourney. Actually, I realized after the fact, I went back and watched things. It's nice because the preview that Microsoft gives you inside of Copilot for Microsoft Edge for the browser, the DALL-E generation that you get out of that platform is actually DALL-E 3. It's the latest version of DALL-E, so that's pretty cool that you get that in the preview mode.
GEORGE: I haven't watched the podcast yet. Was there a winner?
SANTI: It was so hard. Here's where we ended. We struggled with it. There was not a winner because they were both exceptionally amazing, but they were different in their own way. That's why it was too difficult to choose a winner. We did pull up and we displayed the results, one result from each side by side so that the audience can go ahead and chime in, in the comment section when they see it. My takeaway was it's hard for me to say who win because they were both so good, but they were so different.
I think that's what's going to happen from now on is people in marketing or people in the design and a design role, they're going to go, "I'm not going to stick to one platform. I'm going to have two or three and give it the same prompt because there's variations or differences between them and not have more options to choose from." They were just so good. The images were so exceptionally good that it was hard to choose a winner honestly.
GEORGE: The speed again of change, right?
SANTI: Oh yes.
GEORGE: There's a lot of trigger points in here where major things happened that helped in advance. Again, it feels, if you think about that, the Gartner hype curve, right?
SANTI: Yes.
GEORGE: I was at Gartner symposium a couple of weeks ago and they talked about us getting close to the peak of that hype curve, that hype curve from a progress standpoint. Usually, when you fall off and it goes into disillusionment and people haven't really been able to implement it or get the business value out of a certain technology right and then it ramps back up again, I don't know that we're going to hit that trough with this because the usefulness and the speed of change are having multiple tools like we just talked about does give you choice.
At some point, organizations might choose one over the other or come up with a model to aggregate and enter prompts and get results from two or three at the same time and be able to be the human in the interaction and select from the outcomes and choose the path forward, which on one day might be with one tool and another day might be with another tool.
That does allow the world and these developments to move forward without all converging either, right? It'll all converge then there's no differentiation. We get this group thing going that that's not valuable because you narrow the set of potential outcomes. You want to broaden the set of potential outcomes from all of these standpoints, whether using it for written content or graphical content or data analysis or code development or whatever it is.
SANTI: You know what? For example, OpenAI now has an enterprise-level agreement for ChatGPT. I can see all these other platforms doing that where they're just going to say, "Hey, look, we're going to give you more features, more capacity, more speed, more prioritization, more results if you sign up for this." I can see that happening across the board where all the different types of AI platforms are going to eventually spin up.
To your point and depending on the company's philosophy, I think you sign up for multiple ones because you're going to get different results. Sometimes you may want to combine stuff from one and the other and make something that's very much unique in yours. DALL-E, I'm enjoying it. I know that Sam really likes Midjourney, but he's actually entertaining using the pay-subscription version of DALL-E now after seeing the results.
All right, and here we are, homestretch 2022. This thing called "Stable Diffusion," the Stable Diffusion is really more about, again, using deep learning to generate images that were based on text description. It got really good at the prompt. This is what made prompts so, so successful using natural language. It just got better at it. It stabilized that whole process. Here we are, 2023. We got ChatGPT on its fourth version. We have DALL-E on its third. We have Microsoft Copilot rolling out like crazy, shaking up the entire Microsoft world.
This is where we're at. All this really has led to where we are today, which is that green box at the end there, which is AI that's able to generate very unique content and unique results based on the input we give it. Man, this is a great graph. He really thought through this. I love seeing some of these names that I haven't heard, things like AlphaGo and even the VAEs. I haven't heard that in so long.
It's nice to take a step back in. Honestly, George, I walked through this. While I walk out at a high level in my mind, my head spinning because it reminds me of how far we've come. Can I tell you something? This is just the tip of the iceberg. If we think that these last 10 years is what defines AI, no, it's what defines AI as far as we're at today. This is going to happen so fast that within 12 months, we'll be talking about stuff that we've only dreamed of.
By the way, that's the thing. The speed in which AI is developing is because we have all these building blocks in place. Trust me. There'll be more building blocks that are going to be added to this matrix. My only hope is that they don't start regulating it to the point where they slow down the speed of progress. That's the thing that I really hope that doesn't come to that.
GEORGE: That's an interesting point. The part of the discussions at Gartner a couple of weeks ago were around two things. I saw two distinct approaches to leveraging AI platforms. One was they either had their own platform that they were trying to promote or they were using a single platform, right? That was one of the threads. The second thread was what we talked about a little bit ago, which was aggregating multiple LLMs, using them in unison generally with a private data lake, right?
That was storing your data and interacting, right? This leads to regulation. We've talked on previous podcasts really around what's regulation going to look like, what should be included in it, should industries or other constituencies get together to form their own regulation. Those were the things we were talking about. What I heard consistently in some very specific threads at Gartner were, "How do you survive an audit," right?
SANTI: Yes, that's a good point. [laughs]
GEORGE: The regulation is going to happen. To your point, the hope is it's not so onerous that it stifles development or use or anything like that. People do need to start to think about what are they going to do with the data in their environment. How are they going to use it? How are they going to survive an audit effectively? Will they be able to prove what they did, how they did it, what data was used, where was the data stored, all of those things that happen behind the scenes that will effectively be regulated, right? The ability to track and trace what actually happened to get you there seems like it's emerging as the more important thing than the regulation itself, right?
SANTI: Yes, the transparency piece, the accountability piece. Yes, explaining how you generate it, what you generated, where they originate from. All right. Well, listen, I could talk about AI for another hour, George, but I can't, [laughs] so our producers will not allow us to do so. We have to bring this podcast to an end. Folks, thank you for joining us on this journey, this 10-year journey of AI.
This is the one episode that's going to be the launchpad for a lot of AI discussions coming in the future, right? We just wanted to share with you all how we got to where we got. Thank you for joining us. This does bring our podcast to an end. This is a good time to remind you all to subscribe to Tech UNMUTED on your favorite podcast platform. In fact, do it right now. Right now. I'm not leaving until you do. Folks, thank you very much. Till next time, stay curious, stay connected. See you all next time.
CLOSING VOICEOVER: Visit www.fusionconnect.com/techunmuted for show notes and more episodes. Thanks for listening.
Episode Credits:
Produced by: Fusion Connect
Listen on Your Favorite Podcast Player:
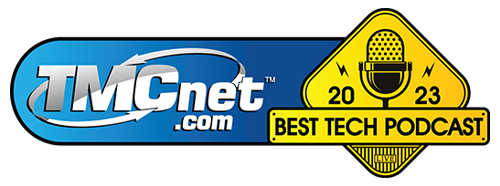
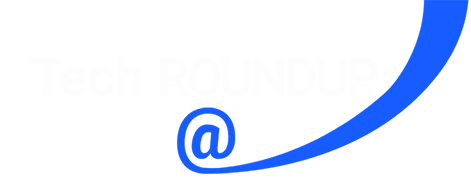
Expert insights, exclusive content, and the latest updates on Microsoft products and services - direct to your inbox. Subscribe to Tech ROUNDUP!
Tech UNMUTED, the podcast of modern collaboration, where we tell the stories of how collaboration tools enable businesses to be more efficient and connected. Humans have collaborated since the beginning of time – we’re wired to work together to solve complex problems, brainstorm novel solutions and build a connected community. On Tech UNMUTED, we’ll cover the latest industry trends and dive into real-world examples of how technology is inspiring businesses and communities to be more efficient and connected. Tune in to learn how today's table-stakes technologies are fostering a collaborative culture, serving as the anchor for exceptional customer service.
Get show notes, transcripts, and other details at www.fusionconnect.com/techUNMUTED. Tech UNMUTED is a production of Fusion Connect, LLC.